The ability to logically and efficiently organize risk data is a critical success factor and a backbone for effective decision-making, strategic planning, and operational efficiency.
Achieving effective data management often presents a unique set of challenges, especially when many stakeholders across multiple business units are all looking for the most up-to-date real-time view of the company's data.
Many firms try to adopt a top-down data management approach but can face limitations in how this approach is adhered to and followed. However, when implemented, this approach often falls short, resulting in a patchwork of governance structures that make collaboration and workflow management slow and inefficient.
Improving Data Governance for Catastrophe Risk
For an insurance industry swimming in data, it can sometimes feel as if firms are drowning in it.
In larger brokers and reinsurers with hundreds of underwriters, exposure managers, cat modelers, and portfolio managers, each individual is responsible for a significant amount of exposure and loss analytics data. They are also responsible for adhering to the company's data file naming conventions and policies as part of their day-to-day responsibilities.
During peak periods in the insurance calendar such as renewals, insurers, reinsurers, or brokers are likely to conduct multiple analyses against a portfolio or sub-section of a portfolio, resulting in multiple copies of results.
These governance structures and policies are then tested as firms can experience exponential growth in the number of exposures and results.
Reinsurance and broker cat modelers are often running dozens of cedents or exposures within a few weeks, and exploring multiple model analyses for each of them.
This can quickly escalate into managing dozens of copies of exposures and results, all without an effective way to organize them, resulting in significant time and expense trying to locate the right portfolio to analyze and share with stakeholders.
When companies operate their data in silos, this difficulty is exacerbated, as isolated data repositories inhibit a unified, holistic view of the organization's information.
While data tagging isn’t new to the industry, the ability to create and enforce a firm-wide governance structure is often constrained by application design and the IT environment.
Data tagging, a tool that can help firms search for and manage risks, is often limited to a single application designed for a distinct function.
As a risk moves along each function in the risk lifecycle (from underwriting to exposure management to catastrophe modeling), the naming governance or tags created by one discipline in one app often do not carry to another.
As a result, each discipline creates its governance strategy, making it increasingly difficult to collaborate with risk stakeholders.
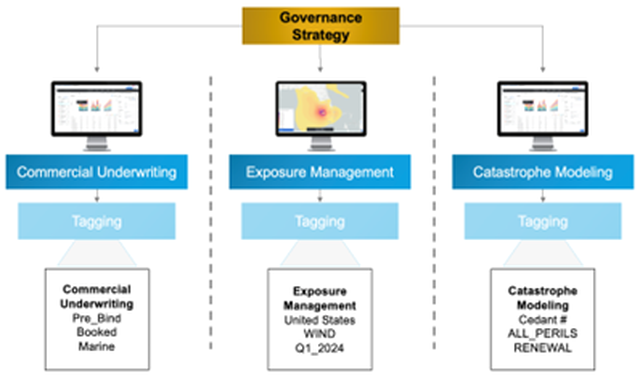
Moody’s Vision for Better Governance of Risk Data
Our vision to solve these challenges and transform the way companies manage their data is a simple yet powerful capability that can significantly enhance data organization and automation – through the use of cross-app data tagging.
In December 2023, Moody's released the very first step in this vision within Risk Modeler™, our cloud-native catastrophe modeling application, by providing users with the ability to assign labels or tags to data assets; such as exposure information, model profiles, and loss results.
Using these flexible tags, users can create custom data groupings following their company data organization guidelines, to help categorize and structure data in a more coherent and accessible way.
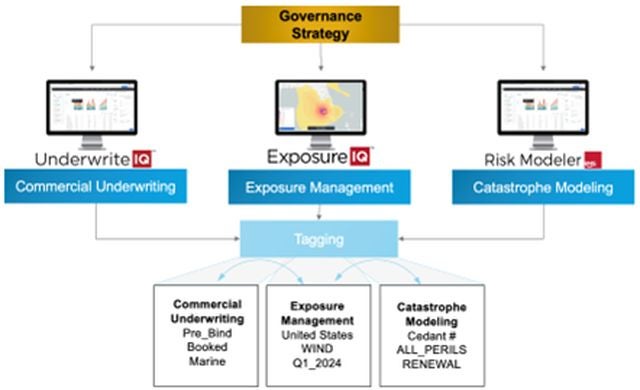
Tagging not only improves data organization but also opens up new possibilities for automation and action-taking against that data. In the future, Risk Modeler and other applications on the Intelligent Risk Platform™ will be able to leverage data tagging to help streamline event response and other cross-application workflows.
Because applications hosted on the platform share a unified data store for exposure and results data, tagging a risk or a portfolio seamlessly carries over from one application to another.
With this ability, we see multiple workflows being dramatically streamlined. For example:
- By tagging data related to specific perils, regions, or other triggers, companies can automate their response mechanisms, reducing manual intervention and increasing the speed and efficiency of their reactions.
- Data tagging can be instrumental in speeding up the time it takes to validate a new or updated model. Tagging data with exposure to certain models allows companies to quickly identify which portfolios need to be run against the new model version, track changes, maintain version control, and identify drivers of change more effectively. This can lead to faster model adoption, giving companies a competitive advantage.
- Data tagging can also facilitate data archiving. Auto-archiving based on tags can ensure that data is stored efficiently and can be easily retrieved when needed. This can significantly reduce the time and resources spent on data management, freeing up these resources for more strategic tasks.
- Establishing proper tagging guidelines for primary underwriters helps automate the process of adding new accounts to existing portfolios when new business is booked and removing expired policies. This makes it easier for portfolio managers and cat modelers to have access to a real-time view of the company's exposure.
Data Governance for Improved Collaboration
Data tagging alone will not solve your firm-wide data governance strategy, rather it is a tool that can help establish and maintain rules across the organization. Implementing a successful data governance strategy requires strong leadership, careful consideration of the right type of naming and tagging parameters, and adherence by your organization.
To learn more about how to think about this strategy you can read our blog on the topic.
Data tagging is just another example of how Moody’s is trying to reduce friction within the risk lifecycle. Enabling greater efficiencies in data organization and automation can enhance the way firms handle their data, leading to more effective decision-making, improved operational efficiency, and ultimately, business success.
To learn more about how we are helping firms solve some of the most complex data collaboration challenges, such as data schema management, visit the Digitizing Workflows page.