Risk analytics has become increasingly complex. Many of our clients are looking to extend the breadth of available analytics by accessing Moody’s RMS models and models developed by other vendors.
But to implement risk analytics using models from multiple vendors, clients often find themselves drowning in the complexity, with issues ranging from data preparation and data conversion, systems and workflow integration, through to infrastructure plumbing.
To address the complexity, Moody’s RMS Intelligent Risk Platform™ is designed from the ground up, unifying models, data, and applications to simplify these repetitive data, systems, and workflow problems that each client must currently solve within their own IT infrastructure and systems.
One of the biggest challenges to overcome when using and integrating models developed by multiple vendors is to get exposure data in its various formats (EDM, MRI, OED, CEDE) converted and mapped into a schema that a model vendor supports.
This may sound simple, however, each of these complex data schemas has its own data representation and attribute encoding. The key to getting the best out of a given model is ensuring each model’s native exposure format and the completeness of the input are used within a model execution.
If these data conversions and mappings are not done correctly, any analyses performed using these models would suffer from a ‘garbage-in-garbage-out’ problem.
There is a risk that the benefits of expanding analytical breadth by integrating models from various vendors can be outweighed by the challenges of mapping the data correctly.
Common Data Schema
Moody’s RMS Intelligent Risk Platform (IRP) looks to address these challenges by defining and utilizing a common data schema and also by providing services to map data from different industry standard schemas into the common schema.
This means that clients using the IRP do not need to spend valuable resources to develop and maintain complex data mappings capabilities, and can simply focus resources on utilizing the power of their newly expanded analytical breadth.
The foundation of the IRP consists of common exposure and loss data stores, built on a logical data model and a data specification that is used to express and exchange risks.
The platform also provides the necessary services to enable users to import and transform exposure data stored in CEDE (Catastrophe Exposure Database Exchange) and OED (Open Exposure Data) schemas.
By utilizing these data import and transformation services, not only can clients access all Moody’s RMS catastrophe models but also access models developed by other vendors that have been integrated through the platform, without the need to map the exposure data in multiple different schemas.
So why is mapping exposure information from one data schema to another data schema so hard?
Many who have attempted developing in-house capabilities have told us that they find themselves drowning in complications, both in developing and maintaining these mappings, as different schemas capture information about the same exposure in different ways.
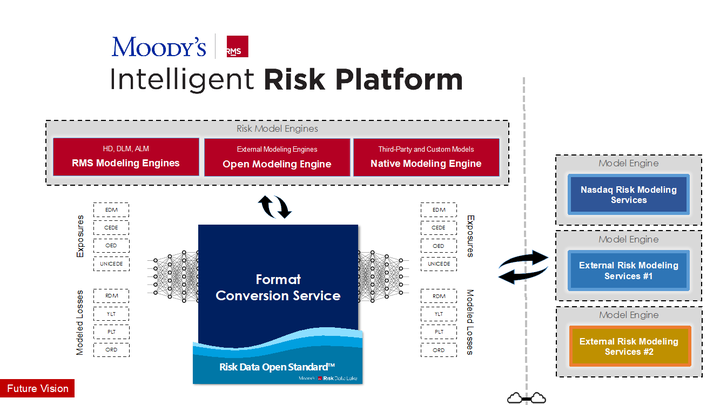
Take the peril code as an example. All three major schemas – CEDE, OED, and the EDM, capture the peril information differently.
In the CEDE schema, peril, sub-peril, and all combinations are coded into a numeric code resulting in over 1.6 million possible values.
With the Exposure Data Module (EDM), the main code is for the top-level perils while the sub-peril information is captured during the model run in other tables. In order to get the accurate mapping of exposure, it is critical to get the peril information mapped correctly between these schemas.
Another challenge in exposure mapping is the use of different code values, such as the construction code or occupancy code, between the various schemas. Values for such code fields along with many secondary modifiers, are different between all three exposure schemas.
There are also instances where even within the same exposure schema, there are variations in values for the same field by region and peril because multiple vendors have developed with different encoding assumptions.
One example is in earthquake models: the set of values defined for North America earthquake might be different than the values used for Japan earthquake. Mappings must be defined and maintained for all such fields and peril/region variations.
Lastly, there are differences among schemas in how financial information is captured. For example, in one schema the total insured value is captured within one data row with different columns for building versus content values.
In another schema, the same information is captured in multiple data rows with a code value distinguishing the data row containing the building value from the one containing the content value.
Achieving High-Fidelity Data Mapping
Similar differences exist for other financial details such as deductibles and limit values. All these details need to be incorporated to achieve high-fidelity mapping and avoid ‘garbage-in-garbage-out’ issues.
The mapping services deployed in Moody’s RMS Intelligent Risk Platform are designed to achieve this high-fidelity mapping between the various exposure schemas and their various versions.
The high level of fidelity is achieved through rigorous testing of the mapping engines by exposing them to many datasets and fine-tuning the mapping logic. Mapping engines produce multiple reports comparing the key aspects of the exposure data between the source and the destination data schemas to handle cases such as the ones above.
With the common exposure and loss store at its core and the services to map data from various schemas, the platform makes it easy for clients to access models not only developed by Moody’s RMS but also by multiple other vendors.
It delivers a solution for clients to extend the breadth of their analytics and overcomes the complications of data preparation and data conversion, system integration, and infrastructure plumbing, which hold back multi-vendor model analysis.
For more information about this announcement and to participate in the preview program, clients can reach out to their Moody’s RMS representatives or email us at info@rms.com