Tag: NORTH AMERICA
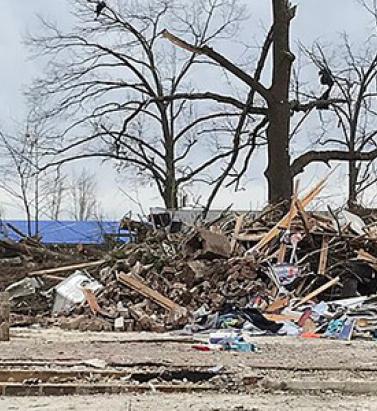
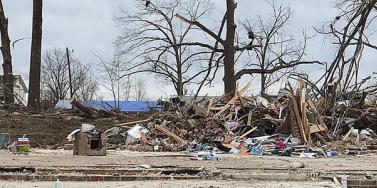
Severe Convective Storms: Experience Cannot Tell the Whole Story
May 05, 2020Severe convective storms can strike with little warning across vast areas of the planet, yet some insurers still rely solely on historical records that do not capture the full spectrum of risk at given locations. EXPOSURE explores the limitations of this approach and how they can be overcome with cat modeling Attritional and high-severity claims from severe convective storms (SCS) — tornadoes, hail, straight-line winds and lightning — are on the rise. In fact, in the U.S., average annual insured losses (AAL) from SCS now rival even those from hurricanes, at around US$17 billion, according to the latest RMS U.S. SCS Industry Loss Curve from 2018. In Canada, SCS cost insurers more than any other natural peril on average each year. Despite the scale of the threat, it is often overlooked as a low volatility, attritional peril Christopher Allen RMS “Despite the scale of the threat, it is often overlooked as a low volatility, attritional peril,” says Christopher Allen, product manager for the North American SCS and winterstorm models at RMS. But losses can be very volatile, particularly when considering individual geographic regions or portfolios (see Figure 1). Moreover, they can be very high. “The U.S. experiences higher insured losses from SCS than any other country. According to the National Weather Service Storm Prediction Center, there over 1,000 tornadoes every year on average. But while a powerful tornado does not cause the same total damage as a major earthquake or hurricane, these events are still capable of causing catastrophic losses that run into the billions.” Figure 1: Insured losses from U.S. SCS in the Northeast (New York, Connecticut, Rhode Island, Massachusetts, New Hampshire, Vermont, Maine), Great Plains (North Dakota, South Dakota, Nebraska, Kansas, Oklahoma) and Southeast (Alabama, Mississippi, Louisiana, Georgia). Losses are trended to 2020 and then scaled separately for each region so the mean loss in each region becomes 100. Source: Industry Loss Data Two of the costliest SCS outbreaks to date hit the U.S. in spring 2011. In late April, large hail, straight-line winds and over 350 tornadoes spawned across wide areas of the South and Midwest, including over the cities of Tuscaloosa and Birmingham, Alabama, which were hit by a tornado rating EF-4 on the Enhanced Fujita (EF) scale. In late May, an outbreak of several hundred more tornadoes occurred over a similarly wide area, including an EF-5 tornado in Joplin, Missouri, that killed over 150 people. If the two outbreaks occurred again today, according to an RMS estimate based on trending industry loss data, each would easily cause over US$10 billion of insured loss. However, extreme losses from SCS do not just occur in the U.S. In April 1999, a hailstorm in Sydney dropped hailstones of up to 3.5 inches (9 centimeters) in diameter over the city, causing insured losses of AU$5.6 billion according to the Insurance Council of Australia (ICA), currently the most costly insurance event in Australia’s history [1]. “It is entirely possible we will soon see claims in excess of US$10 billion from a single SCS event,” Allen says, warning that relying on historical data alone to quantify SCS (re)insurance risk leaves carriers underprepared and overexposed. Historical Records are Short and Biased According to Allen, the rarity of SCS at a local level means historical weather and loss data fall short of fully characterizing SCS hazard. In the U.S., the Storm Prediction Center’s national record of hail and straight-line wind reports goes back to 1955, and tornado reports date back to 1950. In Canada, routine tornado reports go back to 1980. “These may seem like adequate records, but they only scratch the surface of the many SCS scenarios nature can throw at us,” Allen says. “To capture full SCS variability at a given location, records should be simulated over thousands, not tens, of years,” he explains. “This is only possible using a cat model that simulates a very wide range of possible storms to give a fuller representation of the risk at that location. Observed over tens of thousands of years, most locations would have been hit by SCS just as frequently as their neighbors, but this will never be reflected in the historical records. Just because a town or city has not been hit by a tornado in recent years doesn’t mean it can’t be.” To capture full SCS variability at a given location, records should be simulated over thousands, not tens, of years Shorter historical records could also misrepresent the severity of SCS possible at a given location. Total insured catastrophe losses in Phoenix, Arizona, for example, were typically negligible between 1990 and 2009, but on October 5, 2010, Phoenix was hit by its largest-ever tornado and hail outbreak, causing economic losses of US$4.5 billion. (Source: NOAA National Centers for Environmental Information) Just like the national observations, insurers’ own claims histories, or industry data such as presented in Figure 1, are also too short to capture the full extent of SCS volatility, Allen warns. “Some primary insurers write very large volumes of natural catastrophe business and have comprehensive claims records dating back 20 or so years, which are sometimes seen as good enough datasets on which to evaluate the risk at their insured locations. However, underwriting based solely on this length of experience could lead to more surprises and greater earnings instability.” If a Tree Falls and No One Hears… Historical SCS records in most countries rely primarily on human observation reports. If a tornado is not seen, it is not reported, which means that unlike a hurricane or large earthquake it is possible to miss SCS in the recent historical record. “While this happens less often in Europe, which has a high population density, missed sightings can distort historical data in Canada, Australia and remote parts of the U.S.,” Allen explains. Another key issue is that the EF scale rates tornado strength based on how much damage is caused, but this does not always reflect the power of the storm. If a strong tornado occurs in a rural area with few buildings, for example, it won’t register high on the EF scale, even though it could have caused major damage to an urban area. “This again makes the historical record very challenging to interpret,” he says. “Catastrophe modelers invest a great deal of time and effort in understanding the strengths and weaknesses of historical data. By using robust aspects of observations in conjunction with other methods, for example numerical weather simulations, they are able to build upon and advance beyond what experience tells us, allowing for more credible evaluation of SCS risk than using experience alone.” Then there is the issue of rising exposures. Urban expansion and rising property prices, in combination with factors such as rising labor costs and aging roofs that are increasingly susceptible to damage, are pushing exposure values upward. “This means that an identical SCS in the same location would most likely result in a higher loss today than 20 years ago, or in some cases may result in an insured loss where previously there would have been none,” Allen explains. Calgary, Alberta, for example, is the hailstorm capital of Canada. On September 7, 1991, a major hailstorm over the city resulted in the country’s largest insured loss to date from a single storm: CA$343 million was paid out at the time. The city has of course expanded significantly since then (see Figure 2), and the value of the exposure in preexisting urban areas has also increased. An identical hailstorm occurring over the city today would therefore cause far larger insured losses, even without considering inflation. Figure 2: Urban expansion in Calgary, Alberta, Canada. European Space Agency. Land Cover CCI Product User Guide Version 2. Tech. Rep. (2017). Available at: maps.elie.ucl.ac.be/CCI/viewer/download/ESACCI-LC-Ph2-PUGv2_2.0.pdf “Probabilistic SCS cat modeling addresses these issues,” Allen says. “Rather than being constrained by historical data, the framework builds upon and beyond it using meteorological, engineering and insurance knowledge to evaluate what is physically possible today. This means claims do not have to be ‘on-leveled’ to account for changing exposures, which may require the user to make some possibly tenuous adjustments and extrapolations; users simply input the exposures they have today and the model outputs today’s risk.” The Catastrophe Modeling Approach In addition to their ability to simulate “synthetic” loss events over thousands of years, Allen argues, cat models make it easier to conduct sensitivity testing by location, varying policy terms or construction classes; to drill into loss-driving properties within portfolios; and to optimize attachment points for reinsurance programs. SCS cat models are commonly used in the reinsurance market, partly because they make it easy to assess tail risk (again, difficult to do using a short historical record alone), but they are currently used less frequently for underwriting primary risks. There are instances of carriers that use catastrophe models for reinsurance business but still rely on historical claims data for direct insurance business. So why do some primary insurers not take advantage of the cat modeling approach? “Though not marketwide, there can be a perception that experience alone represents the full spectrum of SCS risk — and this overlooks the historical record’s limitations, potentially adding unaccounted-for risk to their portfolios,” Allen says. What is more, detailed studies of historical records and claims “on-leveling” to account for changes over time are challenging and very time-consuming. By contrast, insurers who are already familiar with the cat modeling framework (for example, for hurricane) should find that switching to a probabilistic SCS model is relatively simple and requires little additional learning from the user, as the model employs the same framework as for other peril models, he explains. A US$10 billion SCS loss is around the corner, and carriers need to be prepared and have at their disposal the ability to calculate the probability of that occurring for any given location Furthermore, catastrophe model data formats, such as the RMS Exposure and Results Data Modules (EDM and RDM), are already widely exchanged, and now the Risk Data Open Standard™ (RDOS) will have increasing value within the (re)insurance industry. Reinsurance brokers make heavy use of cat modeling submissions when placing reinsurance, for example, while rating agencies increasingly request catastrophe modeling results when determining company credit ratings. Allen argues that with property cat portfolios under pressure and the insurance market now hardening, it is all the more important that insurers select and price risks as accurately as possible to ensure they increase profits and reduce their combined ratios. “A US$10 billion SCS loss is around the corner, and carriers need to be prepared and have at their disposal the ability to calculate the probability of that occurring for any given location,” he says. “To truly understand their exposure, risk must be determined based on all possible tomorrows, in addition to what has happened in the past.” [1] Losses normalized to 2017 Australian dollars and exposure by the ICA. Source: https://www.icadataglobe.com/access-catastrophe-data. To obtain a holistic view of severe weather risk contact the RMS team here
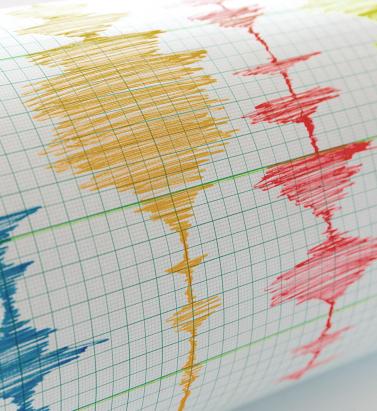
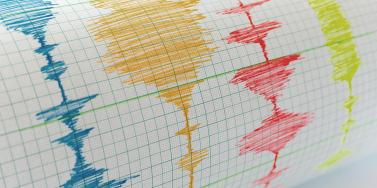
An Unparalleled View of Earthquake Risk
March 17, 2017As RMS launches Version 17 of its North America Earthquake Models, EXPOSURE looks at the developments leading to the update and how distilling immense stores of high-resolution seismic data into the industry’s most comprehensive earthquake models will empower firms to make better business decisions. The launch of RMS’ latest North America Earthquake Models marks a major step forward in the industry’s ability to accurately analyze and assess the impacts of these catastrophic events, enabling firms to write risk with greater confidence due to the underpinning of its rigorous science and engineering. The value of the models to firms seeking new ways to differentiate and diversify their portfolios as well as price risk more accurately, comes from a host of data and scientific updates. These include the incorporation of seismic source data from the U.S. Geological Survey (USGS) 2014 National Seismic Hazard Mapping Project. First groundwater map for Liquefaction “Our goal was to provide clients with a seamless view of seismic hazards across the U.S., Canada and Mexico that encapsulates the latest data and scientific thinking— and we’ve achieved that and more,” explains Renee Lee, head of earthquake model and data product management at RMS. “There have been multiple developments – research and event-driven – which have significantly enhanced understanding of earthquake hazards. It was therefore critical to factor these into our models to give our clients better precision and improved confidence in their pricing and underwriting decisions, and to meet the regulatory requirements that models must reflect the latest scientific understanding of seismic hazard.” Founded on Collaboration Since the last RMS model update in 2009, the industry has witnessed the two largest seismic-related loss events in history – the New Zealand Canterbury Earthquake Sequence (2010-2011) and the Tohoku Earthquake (2011). “We worked very closely with the local markets in each of these affected regions,” adds Lee, “collaborating with engineers and the scientific community, as well as sifting through billions of dollars of claims data, in an effort not only to understand the seismic behavior of these events, but also their direct impact on the industry itself.” A key learning from this work was the impact of catastrophic liquefaction. “We analyzed billions of dollars of claims data and reports to understand this phenomenon both in terms of the extent and severity of liquefaction and the different modes of failure caused to buildings,” says Justin Moresco, senior model product manager at RMS. “That insight enabled us to develop a high-resolution approach to model liquefaction that we have been able to introduce into our new North America Earthquake Models.” An important observation from the Canterbury Earthquake Sequence was the severity of liquefaction which varied over short distances. Two buildings, nearly side-by-side in some cases, experienced significantly different levels of hazard because of shifting geotechnical features. “Our more developed approach to modeling liquefaction captures this variation, but it’s just one of the areas where the new models can differentiate risk at a higher resolution,” said Moresco. The updated models also do a better job of capturing where soft soils are located, which is essential for predicting the hot spots of amplified earthquake shaking.” “There is no doubt that RMS embeds more scientific data into its models than any other commercial risk modeler,” Lee continues. “Throughout this development process, for example, we met regularly with USGS developers, having active discussions about the scientific decisions being made. In fact, our model development lead is on the agency’s National Seismic Hazard and Risk Assessment Steering Committee, while two members of our team are authors associated with the NGA-West 2 ground motion prediction equations.” The North America Earthquake Models in Numbers 360,000 Number of fault sources included in the UCERF3, the USGS California seismic source model >3,800 Number of unique U.S. vulnerability functions in RMS’ 2017 North America Earthquake Models for building shake coverage, with the ability to further differentiate risk based on 21 secondary building characteristics >30 Size of team at RMS that worked on updating the latest model Distilling the Data While data is the foundation of all models, the challenge is to distil it down to its most business-critical form to give it value to clients. “We are dealing with data sets spanning millions of events,” explains Lee, “for example, UCERF3 — the USGS California seismic source model — alone incorporates more than 360,000 fault sources. So, you have to condense that immense amount of data in such a way that it remains robust but our clients can run it within ‘business hours’.” Since the release of the USGS data in 2014, RMS has had over 30 scientists and engineers working on how to take data generated by a super computer once every five to six years and apply it to a model that enables clients to use it dynamically to support their risk assessment in a systematic way. “You need to grasp the complexities within the USGS model and how the data has evolved,” says Mohsen Rahnama, chief risk modeling officer and general manager of the RMS models and data business. “In the previous California seismic source model, for example, the USGS used 480 logic tree branches, while this time they use 1,440 logic trees. You can’t simply implement the data – you have to understand it. How do these faults interact? How does it impact ground motion attenuation? How can I model the risk systematically?” As part of this process, RMS maintained regular contact with USGS, keeping them informed of how they were implementing the data and what distillation had taken place to help validate their approach. Building Confidence Demonstrating its commitment to transparency, RMS also provides clients with access to its scientists and engineers to help them drill down in the changes into the model. Further, it is publishing comprehensive documentation on the methodologies and validation processes that underpin the new version. Expanding the Functionality Upgraded soil amplification methodology that empowers (re)insurers to enter a new era of high-resolution geotechnical hazard modeling, including the development of a Vs30 (average shear wave velocity in the top 30 meters at site) data layer spanning North America Advanced ground motion models leveraging thousands of historical earthquake recordings to accurately predict the attenuation of shaking from source to site New functionality enabling high and low representations of vulnerability and ground motion 3,800+ unique U.S. vulnerability functions for building shake coverage. Ability to further differentiate risk based on 21 secondary building characteristics Latest modeling for very tall buildings (>40 stories) enables more accurate underwriting of high-value assets New probabilistic liquefaction model leveraging data from the 2010-2011 Canterbury Earthquake Sequence in New Zealand Ability to evaluate secondary perils: tsunami, fire following earthquake and earthquake sprinkler leakage New risk calculation functionality based on an event set includes induced seismicity Updated basin model for Seattle, Mississippi Embayment, Mexico City and Los Angeles. Added a new basin model for Vancouver Latest historical earthquake catalog from the Geological Survey of Canada integrated, plus latest research data on the Mexico Subduction Zone Seismic source data from the U.S. Geological Survey (USGS) 2014 National Seismic Hazard Mapping Project incorporated, which includes the third Uniform California Earthquake Rupture Forecast (UCERF3) Updated Alaska and Hawaii hazard model, which was not updated by USGS
